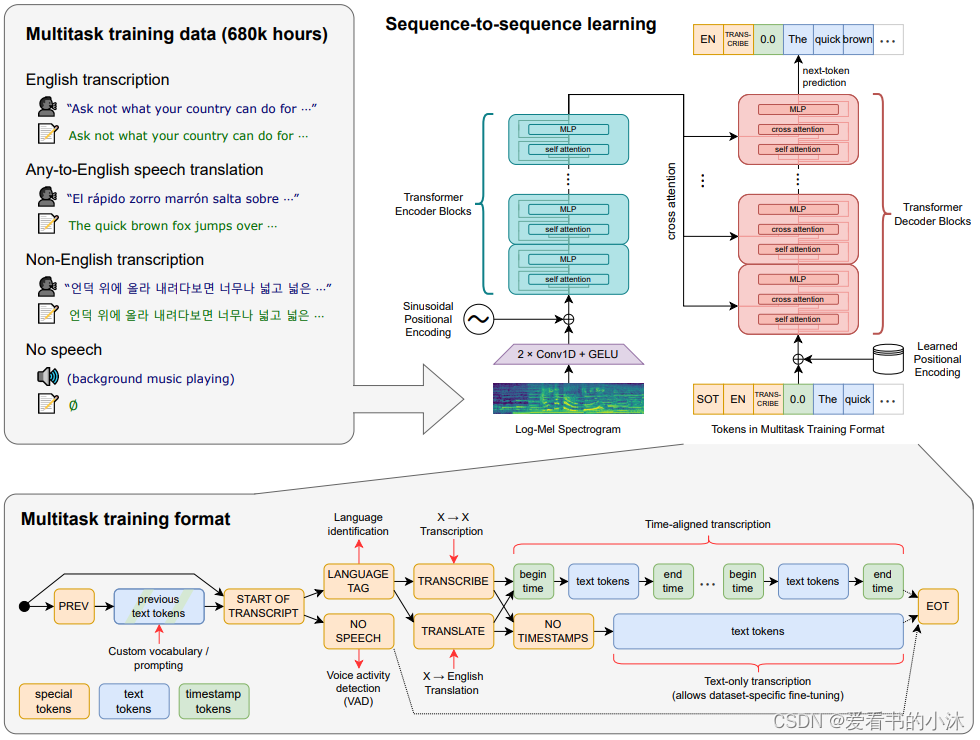
【小沐学AI】Python实现语音识别(whisperX)
Whisper 是一种通用语音识别模型。它是在各种音频的大型数据集上训练的,也是一个多任务模型,可以执行多语言语音识别、语音翻译和语言识别。Transformer 序列到序列模型针对各种语音处理任务进行训练,包括多语言语音识别、语音翻译、口语识别和语音活动检测。这些任务共同表示为解码器要预测的一系列标记,从而允许单个模型取代传统语音处理管道的许多阶段。多任务训练格式使用一组特殊标记作为任务说明符或
1、简介
1.1 whisper
https://arxiv.org/pdf/2212.04356
https://github.com/openai/whisper
Whisper 是一种通用语音识别模型。它是在各种音频的大型数据集上训练的,也是一个多任务模型,可以执行多语言语音识别、语音翻译和语言识别。
Transformer 序列到序列模型针对各种语音处理任务进行训练,包括多语言语音识别、语音翻译、口语识别和语音活动检测。这些任务共同表示为解码器要预测的一系列标记,从而允许单个模型取代传统语音处理管道的许多阶段。多任务训练格式使用一组特殊标记作为任务说明符或分类目标。
1.2 whisperX
https://github.com/m-bain/whisperX
WhisperX:具有单词级时间戳(和Diarization)的自动语音识别
- WhisperX 简介
Whisper 是一个由 OpenAI 开发的通用语音识别模型(ASR),在大量多样化的音频数据集上进行训练,具有惊人的准确性。它同时也是一个多任务模型,可以执行多语言语音识别、语音翻译和语言识别。OpenAI 已经将 Whisper 开源,供社区使用。
WhisperX 是基于 OpenAI 开源项目 Whisper 的一个分支,它是一款功能强大的语音转文本(STT,Speech-to-Text)工具,以其出色的转录能力而闻名,并支持多种语言。更令人兴奋的是,它完全免费。
- 为什么选择 WhisperX
Whisper 在大量多样化的音频数据上进行训练。尽管它能够提供高准确度的转录,但相关的时间戳是话语级别而非单词级别,可能存在数秒不准。而且,Whisper 并不原生支持批处理。
WhisperX 提供了一种快速的自动语音识别方法(在使用 large-v2 时可实现 70x 实时速度),具备单词级时间戳和说话者辨识功能。
- WhisperX的特点
- 使用 whisper large-v2 进行批处理推断,可实现 70x 实时转录。
- 利用 faster-whisper 作为后端,对于 large-v2 只需小于 8GB 的 GPU 显存。(使用beam_size=5)
- 利用 wav2vec2.0 对齐,实现准确的单词级时间戳。
- 利用来自 pyannote-audio 的说话者辨识,实现多说话者 ASR(带有说话者 ID 标签)。
- VAD 预处理,减少幻听问题,并实现无 WER 降低的批处理。
2、安装
2.1 安装cuda
CUDA是什么:CUDA是NVIDIA推出的一种编程技术。它允许开发者使用C语言来编写能在NVIDIA的图形处理器上运行的代码。通过CUDA,开发者可以将GPU用于通用的并行计算,大大提高了计算效率。
CUDA的一个重要特点是,它允许软件开发者直接使用NVIDIA的GPU来执行某些计算密集型的任务。这是因为GPU内部有许多并行处理单元,可以同时执行许多计算任务,从而大大提高了计算速度。
另外,CUDA还提供了一套完整的开发工具链,包括编译器、调试器和性能分析器,使得开发者可以更方便地开发和优化CUDA程序。
首先使用 cmd 命令行输入 nvidia-smi ,在第一行最右边可以看到CUDA的版本号,我的版本是11.6
nvidia-smi
https://pytorch.org/get-started/locally/
打开网址选择一个比较靠近的版本。
选择生成命令为:
pip3 install torch torchvision torchaudio --index-url https://download.pytorch.org/whl/cu118
如果网络不好,可以直接到镜像源进行下载安装 https://download.pytorch.org/whl/torch_stable.html
如果你希望使用 PyTorch 稳定版本,建议使用 CUDA 11.8。可以从 https://developer.nvidia.com/cuda-toolkit-archive 下载相应的存档文件。
验证CUDA是否可用,直接继续命令行中输入 python代码:
import torch
ret = torch.cuda.is_available()
print(ret)
如果输出为True,则代表可以使用GPU显卡了。
import torch
print("Torch version:", torch.__version__)
print("CUDA version:", torch.version.cuda)
print("CUDA available:", torch.cuda.is_available())
if torch.cuda.is_available():
print("CUDA device:", torch.cuda.get_device_name(torch.cuda.current_device()))
device = torch.device('cuda' if torch.cuda.is_available() else 'cpu')
print(device)
https://developer.nvidia.com/cuda-11-8-0-download-archive?target_os=Windows&target_arch=x86_64&target_version=10&target_type=exe_local
nvcc -V
导入 cuDNN:
https://developer.nvidia.com/cudnn-downloads?target_os=Windows&target_arch=x86_64
cuDNN 是一款在 Nvidia CUDA 工具包上运行的深度学习库。它专为加速深度学习任务而设计,提供了在 GPU 上高效执行深度神经网络所需的各种功能和优化。
- 下载 cuDNN。(在登录 Nvidia 账户后,需要注册成为 Nvidia Developer Program 会员。在这个过程中,会要求提供个人信息。)
- 选择CUDA 11.x版本。
- 选择Local Installer for Windows(Zip)下载。
将下载好的 zip 文件解压出来,会得到如下 3 个文件夹:
bin
include
lib
将上述 3 个文件夹移动到CUDA\v11.8目录中,路径为:
C:\Program Files\NVIDIA GPU Computing Toolkit\CUDA\v11.8
添加以下环境变量:
变量名:CUDNN_PATH
变量值:C:\Program Files\NVIDIA GPU Computing Toolkit\CUDA\v11.8
2.2 安装whisperX
pip install git+https://github.com/m-bain/whisperx.git
or
pip install git+https://github.com/m-bain/whisperx.git --upgrade
import whisperx
import gc
import os
device = "cpu" #"cuda"
audio_file = "test.mp3"
batch_size = 16 # reduce if low on GPU mem
compute_type = "int8"#"float16" # change to "int8" if low on GPU mem (may reduce accuracy)
# 1. Transcribe with original whisper (batched)
model = whisperx.load_model("tiny", device, compute_type=compute_type)
# save model to local path (optional)
# model_dir = "/path/"
# model = whisperx.load_model("large-v2", device, compute_type=compute_type, download_root=model_dir)
audio = whisperx.load_audio(audio_file)
result = model.transcribe(audio, batch_size=batch_size)
print(result["segments"]) # before alignment
# delete model if low on GPU resources
# import gc; gc.collect(); torch.cuda.empty_cache(); del model
# 2. Align whisper output
model_a, metadata = whisperx.load_align_model(language_code=result["language"], device=device)
result = whisperx.align(result["segments"], model_a, metadata, audio, device, return_char_alignments=False)
print(result["segments"]) # after alignment
# delete model if low on GPU resources
# import gc; gc.collect(); torch.cuda.empty_cache(); del model_a
import whisperx
import zhconv
from whisperx.asr import FasterWhisperPipeline
import time
class WhisperXTool:
device = "cpu" #"cuda"
audio_file = "test.mp3"
batch_size = 16 # reduce if low on GPU mem
# compute_type = "float16" # change to "int8" if low on GPU mem (may reduce accuracy)
compute_type = "int8" # change to "int8" if low on GPU mem (may reduce accuracy)
fast_model: FasterWhisperPipeline
def loadModel(self):
# 1. Transcribe with original whisper (batched)
self.fast_model = whisperx.load_model("tiny", self.device, compute_type=self.compute_type) #large-v2
print("模型加载完成")
def asr(self, filePath: str):
start = time.time()
audio = whisperx.load_audio(filePath)
result = self.fast_model.transcribe(audio, batch_size=self.batch_size)
s = result["segments"][0]["text"]
s1 = zhconv.convert(s, 'zh-cn')
print(s1)
end = time.time()
print('识别使用的时间:', end - start, 's')
return s1
if __name__ == '__main__':
app = WhisperXTool()
app.loadModel()
app.asr(app.audio_file)
import time
import torch
import os
import whisper
from whisper.utils import get_writer
from faster_whisper import WhisperModel
import whisperx
import gc
def whisper_test():
os.environ['KMP_DUPLICATE_LIB_OK'] = 'TRUE'
filename = "test.mp3"
## window GPU cuda
## window CPU cpu
## mac CPU cpu
## mac GPU
model = whisper.load_model("large-v3",device="cuda")
result = model.transcribe(audio=filename, fp16 =False)
output_directory = "."
word_options = {
"highlight_words": True,
"max_line_count": 50,
"max_line_width": 3
}
srt_writer = get_writer("srt", output_directory)
srt_writer(result, filename, word_options)
def faster_whisper_test():
model_size = "large-v3"
## window cpu
model = WhisperModel(model_size, device="cpu", compute_type="int8", cpu_threads=16)
# window gpu
# model = WhisperModel(model_size, device="cuda", compute_type="float16")
segments, info = model.transcribe("test.mp3", beam_size = 5)
print("Detected language '%s' with probability %f" % (info.language, info.language_probability))
for segment in segments:
print("[%.2fs -> %.2fs] %s" % (segment.start, segment.end, segment.text))
def whisperx_test():
device = "cpu"
model_size = "tiny"
# model_size ="large-v3"
audio_file = "test.mp3"
batch_size = 16
compute_type = "int8"
# widnow CPU
model = whisperx.load_model(model_size, device, compute_type=compute_type)
# window GPU
# model = whisperx.load_model(model_size, "cuda", compute_type="float16")
audio = whisperx.load_audio(audio_file)
result = model.transcribe(audio, batch_size=batch_size)
print(result["segments"])
if __name__ == "__main__":
whisperx_test()
更多AI信息如下:
2024第四届人工智能、自动化与高性能计算国际会议(AIAHPC 2024)将于2024年7月19-21日在中国·珠海召开。
大会网站:更多会议详情
时间地点:中国珠海-中山大学珠海校区|2024年7月19-21日
结语
如果您觉得该方法或代码有一点点用处,可以给作者点个赞,或打赏杯咖啡;
╮( ̄▽ ̄)╭
如果您感觉方法或代码不咋地
//(ㄒoㄒ)//,就在评论处留言,作者继续改进;
o_O???
如果您需要相关功能的代码定制化开发,可以留言私信作者;
(✿◡‿◡)
感谢各位大佬童鞋们的支持!
( ´ ▽´ )ノ ( ´ ▽´)っ!!!
更多推荐
所有评论(0)