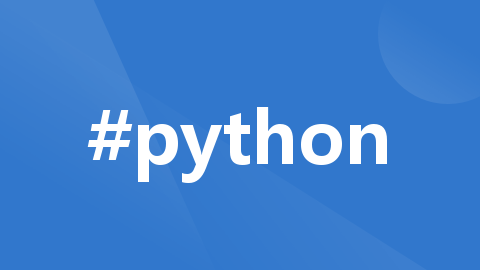
使用 Python 实现遗传算法进行无人机路径规划
遗传算法是一种模拟自然选择过程的优化方法。通过选择、交叉、变异等操作,遗传算法能够逐步优化解的质量,寻找全局最优解。接下来,我们将定义几个类来实现遗传算法,包括无人机模型类和遗传算法控制器类。
·
目录
使用 Python 实现遗传算法进行无人机路径规划
引言
随着无人机技术的快速发展,其在各个领域的应用越来越广泛,尤其是在路径规划方面。遗传算法(GA)作为一种基于自然选择和遗传学原理的优化算法,已经被广泛应用于复杂的优化问题,包括无人机的路径规划。本文将详细介绍如何使用 Python 实现遗传算法进行无人机路径规划,代码将采用面向对象的思想,并提供具体示例。
1. 遗传算法概述
1.1 定义
遗传算法是一种模拟自然选择过程的优化方法。通过选择、交叉、变异等操作,遗传算法能够逐步优化解的质量,寻找全局最优解。
1.2 基本步骤
- 初始化:随机生成初始种群。
- 适应度评估:计算每个个体的适应度,以评估其优劣。
- 选择:根据适应度选择较优个体进入下一代。
- 交叉:通过交叉操作生成新个体。
- 变异:对新个体进行变异,增加多样性。
- 迭代:重复以上步骤,直到满足停止条件(如达到最大代数或适应度达到某一阈值)。
1.3 遗传算法的特点
- 全局搜索能力:遗传算法能够在大搜索空间中找到近似全局最优解。
- 适应性强:可以适应不同的优化问题,具有较强的通用性。
- 并行性:算法的并行特性使其适用于大规模优化问题。
2. 使用 Python 实现遗传算法
2.1 安装必要的库
我们将使用 NumPy 和 Matplotlib 库来实现遗传算法,并进行可视化。确保安装了这些库:
pip install numpy matplotlib
2.2 定义类
接下来,我们将定义几个类来实现遗传算法,包括无人机模型类和遗传算法控制器类。
2.2.1 无人机模型类
无人机模型类用于定义无人机的动态行为及其适应度评估。
import numpy as np
class Drone:
def __init__(self, start_pos, target_pos):
self.start_pos = np.array(start_pos) # 起始位置
self.target_pos = np.array(target_pos) # 目标位置
def calculate_distance(self, path):
"""计算路径的总距离"""
distance = 0
current_pos = self.start_pos
for pos in path:
distance += np.linalg.norm(pos - current_pos) # 距离
current_pos = pos
distance += np.linalg.norm(self.target_pos - current_pos) # 从最后一个点到目标点的距离
return distance
2.2.2 遗传算法类
遗传算法类用于实现路径规划。
import random
class GeneticAlgorithm:
def __init__(self, drone, population_size, mutation_rate, generations):
self.drone = drone
self.population_size = population_size # 种群规模
self.mutation_rate = mutation_rate # 变异率
self.generations = generations # 代数
self.population = self.initialize_population() # 初始化种群
def initialize_population(self):
"""初始化种群"""
population = []
for _ in range(self.population_size):
# 随机生成路径
path = [self.drone.start_pos + np.random.rand(2) * 10 for _ in range(5)] # 随机生成 5 个中间点
population.append(path)
return population
def calculate_fitness(self):
"""计算适应度"""
fitness_scores = []
for path in self.population:
distance = self.drone.calculate_distance(path)
fitness_scores.append(1 / (distance + 1e-6)) # 避免除零错误,适应度与距离成反比
return fitness_scores
def select_parents(self, fitness_scores):
"""选择父母"""
total_fitness = sum(fitness_scores)
selection_probs = [score / total_fitness for score in fitness_scores]
parents_indices = np.random.choice(range(self.population_size), size=2, p=selection_probs)
return [self.population[i] for i in parents_indices]
def crossover(self, parent1, parent2):
"""交叉操作"""
crossover_point = random.randint(1, len(parent1) - 1)
child1 = parent1[:crossover_point] + parent2[crossover_point:]
child2 = parent2[:crossover_point] + parent1[crossover_point:]
return child1, child2
def mutate(self, path):
"""变异操作"""
for i in range(len(path)):
if random.random() < self.mutation_rate:
path[i] = self.drone.start_pos + np.random.rand(2) * 10 # 随机变更路径点
return path
def run(self):
"""运行遗传算法"""
best_path = None
best_distance = float('inf')
for generation in range(self.generations):
fitness_scores = self.calculate_fitness()
new_population = []
for _ in range(self.population_size // 2):
parent1, parent2 = self.select_parents(fitness_scores)
child1, child2 = self.crossover(parent1, parent2)
new_population.append(self.mutate(child1))
new_population.append(self.mutate(child2))
self.population = new_population
# 找到当前代最佳路径
for path in self.population:
distance = self.drone.calculate_distance(path)
if distance < best_distance:
best_distance = distance
best_path = path
return best_path, best_distance
2.3 示例程序
在示例程序中,我们将实现一个简单的无人机路径规划演示。
import matplotlib.pyplot as plt
def main():
start_pos = (0, 0) # 无人机起始位置
target_pos = (10, 10) # 目标位置
drone = Drone(start_pos, target_pos)
ga = GeneticAlgorithm(drone, population_size=100, mutation_rate=0.1, generations=50)
best_path, best_distance = ga.run()
print(f"Best distance: {best_distance}")
# 可视化结果
plt.figure(figsize=(10, 10))
plt.plot([start_pos[0]] + [pos[0] for pos in best_path] + [target_pos[0]],
[start_pos[1]] + [pos[1] for pos in best_path] + [target_pos[1]], 'b-o', label='Path')
plt.scatter(target_pos[0], target_pos[1], label='Target', color='red')
plt.xlim(-1, 12)
plt.ylim(-1, 12)
plt.xlabel('X Position')
plt.ylabel('Y Position')
plt.title('Drone Path Planning using Genetic Algorithm')
plt.legend()
plt.grid()
plt.show()
if __name__ == "__main__":
main()
3. 遗传算法的优缺点
3.1 优点
- 全局优化能力:遗传算法通过群体搜索,可以避免陷入局部最优解。
- 适应性强:适用于多种优化问题,具有良好的通用性。
- 并行性:可以同时处理多个解,适合大规模问题。
3.2 缺点
- 计算复杂性:适应度计算和进化过程可能会导致较高的计算成本。
- 参数调节:算法性能对参数设置(如变异率、种群规模等)敏感,需根据具体问题调整。
- 收敛速度:在某些情况下,遗传算法的收敛速度较慢。
4. 改进方向
为了提升遗传算法的性能,可以考虑以下改进方向:
- 自适应参数调节:根据当前种群的适应度动态调整变异率和选择策略,以提高算法的搜索效率。
- 引入局部搜索:结合局部搜索算法(如爬山算法),在遗传算法的基础上进一步优化个体解。
- 混合算法:将遗传算法与其他优化算法(如粒子群优化、蚁群算法等)结合,利用各自的优点。
5. 应用场景
遗传算法广泛应用于以下领域:
- 无人机路径规划:在复杂环境中优化无人机的飞行路径。
- 机器学习:用于特征选择和模型优化。
- 调度问题:在生产和运输等领域优化资源调度。
结论
遗传算法作为一种强大的优化工具,在无人机路径规划中展现出了其独特的优势。通过 Python 的实现,我们展示了该算法
更多推荐
所有评论(0)